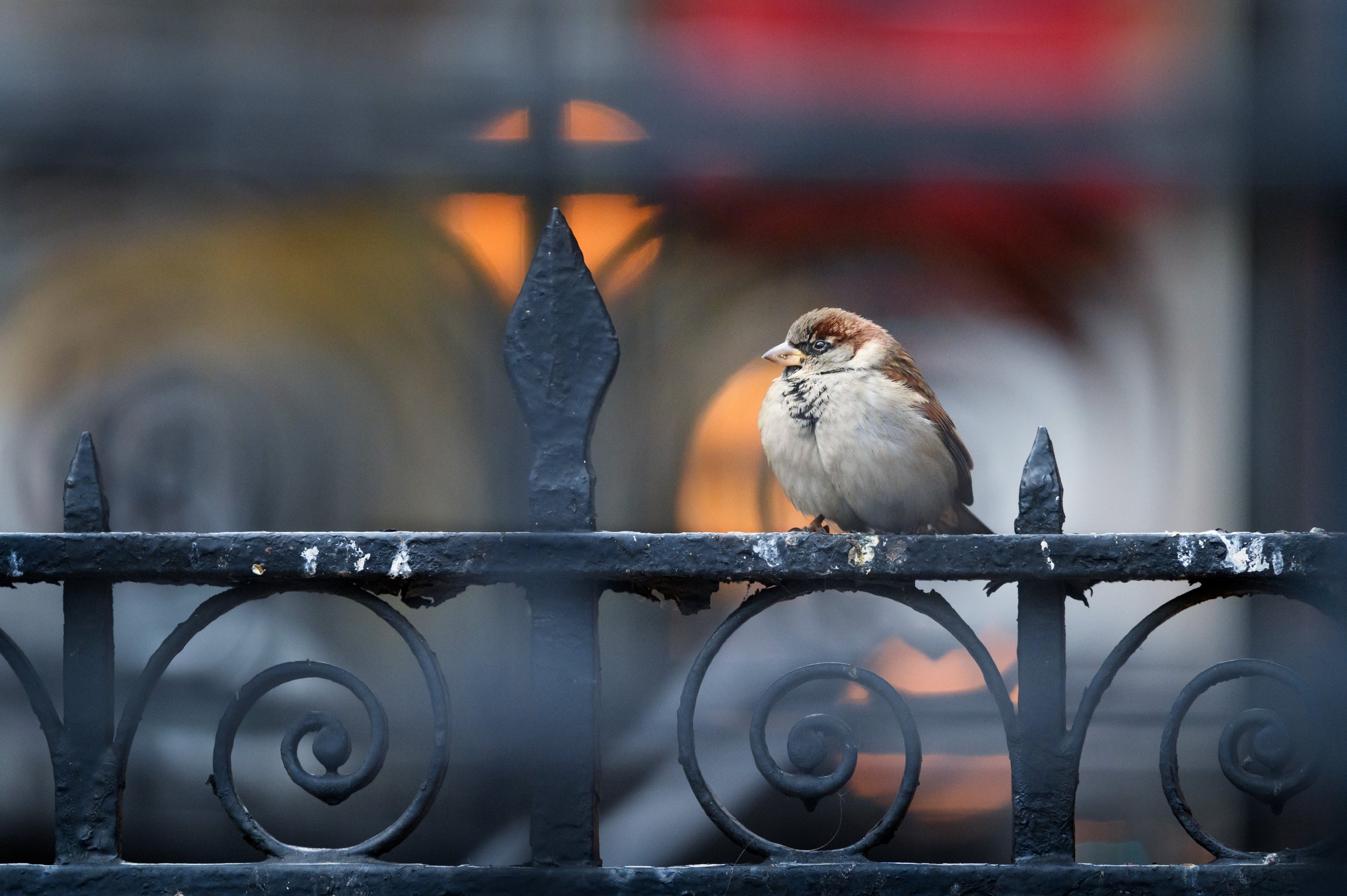
Library
Browse resources published by our research team.
In addition to full texts of our peer-reviewed articles, our library includes research digests that break down our peer-reviewed articles; in-depth reports that thoroughly examine a topic; commentaries that explain the significance of particular issues in Wild Animal Welfare Science; and short communications that briefly survey a field or topic.
Wild Animal Initiative adheres to Open Science TOP Guidelines. Read more here.
Quantifying the neglectedness of wild animal welfare
Michaël Beaulieu's deep dive examines the quality and quantity of mentions of wild animal welfare in the scientific literature and finds it to be a neglected research area.
Questioning the neglectedness of wild animal welfare: passionate claims, illusionary facts, or simple truth?
Wild animal welfare is often suggested to be a neglected research area, worthy of more attention from scientists and policy makers (e.g., Tomasik 2015; Forristal 2022). Considering the general propensity of animal welfare to unleash passions even among scientists (Coghlan & Cardilini 2022), it is important to assess the veracity of these claims. So to objectively assert the need to conduct more research to better understand wild animal welfare and implement sound welfare interventions in the wild, clear evidence (or “stubborn facts” insensitive to people’s inclinations and passions; Adams 1770) supporting the neglectedness of wild animal welfare is required.
Cursory research of the expression “wild animal welfare” in Google Scholar (Nov. 2023) provides 844 outputs, only 7% of which emphasize the expression in their title or abstract. However, such figures may be misleading if wild animal welfare is already considered under alternative synonyms or already incorporated into adjacent disciplines. For instance, the fate of wild animals in response to variable environmental conditions has traditionally been under the scrutiny of conservation practitioners (Brown 2007; Nijhuis 2020). Because conservation studies typically focus on conditions that affect animals in their natural habitat (for instance, environmental conditions changing because of anthropogenic activities and that may be perceived as unpleasant by wild animals), they may theoretically also encompass aspects related to wild animal welfare (Sekar & Shiller 2020). Therefore it is possible wild animal welfare is not so highly neglected, but rather often concealed within existing animal conservation studies. Importantly, because the multidisciplinary field of wild animal welfare includes a variety of approaches, indicators, and taxa, some research areas within this field may be more neglected than others.
Assessing the neglectedness of wild animal welfare
To assess the neglectedness of wild animal welfare, I have conducted a targeted review of the literature in peer-reviewed journals publishing research in animal welfare (three journals: Animal Welfare, Journal of Applied Animal Welfare Science, animal welfare section of Animals) and animal conservation (five journals: Animal Conservation, Biological Conservation, Conservation Biology, Conservation Physiology, Oryx). I selected these journals because of their high visibility and their representativeness in their respective field. I considered a date range of 2013-2022 for the review process, in order to reflect contemporary research on animals. Accordingly, I only included research articles (as opposed to reviews, commentaries) focusing on animals (as opposed to, e.g., plants, policy) in the review of both fields. I identified and classified the study subjects of each welfare science article as “wild” if they were studied in their natural habitat or collected in the wild and then studied in captivity. In conservation articles, I searched for welfare-related terms (e.g., welfare/wellbeing, sentience, emotion, conscious, subjective experience, affective state, compassionate) in both the title and abstract.
Confirming the neglectedness of wild animal welfare
Both the presence of wild animals among all reviewed welfare studies (6%) and the occurrence of welfare-related terms in conservation studies (1%) were very low (Fig. 1). Among welfare-related terms, “welfare/wellbeing” was by far the most commonly used term (90%) in conservation journals. In addition to “welfare/wellbeing,” other welfare-related terms such as “compassionate” (6%) and “sentience” (4%) were also found. However, other welfare-relevant terms such as “conscious,” “subjective experience,” or “affective state” were completely absent. Altogether, these proportions strongly suggest that wild animal welfare is neglected by both the welfare and conservation literature.
Figure 1. Proportion of articles published in three principal animal welfare journals between 2013 and 2022 and including wild animals (left pie, top on mobile), and proportion of articles published in five principle conservation journals over the same period and including welfare-related terms in either their title or abstract (welfare, compassionate and sentience were the only terms found; right panel, bottom on mobile).
Dissecting the neglectedness of wild animal welfare: the example of physiological approaches
To understand how an animal experiences their life in the wild, it is necessary to use approaches that can evaluate and provide evidence of their welfare. Behavioral and physiological markers potentially represent downstream indicators of welfare, and can thus provide insight into the welfare of wild animals (Browning 2022). I focused here on physiological markers specifically, as they may be highly informative (on their own or to complement behavioral approaches) for assessing the welfare of wild animals (see deep dive: Welfare and physiology: a complicated relationship). To quantify the degree to which physiological approaches are also being neglected in wild animal welfare science, I have further examined the welfare science studies previously identified as being conducted on wild animals to determine whether they made use of physiological markers and in which species.
Only 22% of welfare science studies conducted on wild animals included the use of physiological markers (Fig. 2). These results suggest that wildlife biologists either are reluctant to make use of physiological markers in their research relative to other welfare indicators (e.g., living conditions, behavior), or they lack awareness of how to incorporate them. Yet the very existence of the field of conservation physiology, and the numerous studies published, demonstrate that it is possible to measure a diversity of physiological markers in the wild to examine how wild animals are affected by given environmental conditions (Cooke et al. 2013). Nevertheless, despite this potential, thus far such studies have not focused on assessing welfare, as indicated by only 3% of articles published in conservation journals and including physiological parameters mentioning welfare-related terms in their title or abstract. Moreover, the few studies that considered physiological parameters to assess welfare in wild animals focused on a limited number of physiological markers (compared to the vast variety of physiological parameters that could theoretically be measured to assess welfare; e.g., Jerez-Cepa & Ruiz-Jarabo 2021; Whitehead & Dunphy 2022) measured in few taxa, the typical study measuring glucocorticoids in a mammal species (Fig. 2).
Figure 2. Proportion of articles published in three principal animal welfare journals between 2013 and 2022 and including wild animals (left pie, top on mobile) and measuring physiological markers (striped area). The detail of the measured physiological markers (GC: glucocorticoids; H/L: heterophil/lymphocyte ratio, Tº: temperature) and in which taxa (Invert.: invertebrates) they were measured is illustrated in the right pies (bottom on mobile). For clarity, only the first half of the physiological markers measured in articles on wild animal welfare physiology is annotated.
The overrepresentation of glucocorticoids (and their effects on the H/L ratio; Davis et al. 2008) and mammals among studies on the physiology of wild animals is not necessarily surprising, but their measurement is questionable when examining their welfare. Indeed, even though measuring glucocorticoids reflects a general trend observed in animal stress physiology (MacDougall-Shackleton et al. 2019), the relationship between glucocorticoids and welfare remains unclear (Ralph 2016). Therefore, for glucocorticoid measurements to offer useful insights about welfare, they need to be complemented by additional markers (e.g., behavioral observations). Moreover, even though the imbalance in favor of mammals probably reflects the widespread greater interest in these animals, mammals are relatively rare in nature (ca. 0.1% of all animal species and ca. 0.35% of the whole animal biomass on Earth; Mora et al. 2011; Burgin et al. 2018; Bar-On et al. 2018), and the way they experience their lives is probably not comparable to that of other taxa with very different neural organizations (e.g., more common invertebrates; Paul et al. 2020).
Conclusions
Review of recent literature in key peer-reviewed journals in animal welfare showed that the neglectedness of wild animal welfare is indeed a “stubborn fact” and not just a questionable claim. Importantly, the targeted review of journals in animal conservation also provided evidence that this underrepresentation was not simply an artifact of the inclusion of welfare aspects within animal conservation studies (that were found to refer to animal welfare only anecdotally and superficially). Moreover, within the limited number of studies that did focus on wild animal welfare, the limited use of physiological markers further highlights the neglectedness of valuable methodological approaches and taxa. If allowed to persist, such biases will likely limit our understanding of wild animal welfare, as the strength of this field precisely lies in its diversity of approaches and the range of taxa to which they can be applied. Continuing not to take advantage of such potential would be short-sighted.
The limited availability of welfare science studies focusing on wild animals suggests that there may be resistance or barriers to extending the scope of welfare science beyond the traditional context of farmed, companion, and captive-housed animals. A contributing factor may be that assessing welfare is easier in well-known model species maintained under controlled conditions in captivity (e.g., pigs), compared with their wild counterparts living under uncontrollable conditions (e.g., wild boars). Another contributing factor might also be related to the uncertainty about the capacity of many wild animals to have subjective experiences (e.g., invertebrates, but see Anderson & Adolphs 2014; de Waal & Andrews 2022). Despite these inherent challenges, there is increasing evidence that extending the field of animal welfare to wild conditions is feasible (Harvey et al. 2020), particularly through collaborations among scientists from a range of disciplines, and could have far-reaching impacts for our general understanding of how most animals on Earth experience their lives. In addition to advancing general understanding and increasing available knowledge, wild animal welfare studies may also lead to concrete applications — for instance, by informing stakeholders, refining practices, or modifying legislation impacting wildlife. But the full potential of the field of wild animal welfare will be reached only if scientists branch out beyond traditional norms and consider the full range of approaches and taxa that this field has to offer.
Biotelemetry provides insight into a wild eagle’s death
Jane Capozzelli
Jane Capozzelli reviews how biologgers can provide information about welfare, but also pose risks to animals, following the case of a single eagle.
Key takeaways
- Biotelemetry can provide a high level of detail about wild animals’ activities.
- Real-time behavioral and physiological data can indicate how an animal might be feeling.
- Location data can link welfare indicators to the animal’s environmental context.
- Although biotelemetry could advance efforts to improve wild animal welfare, its methods can be harmful or lethal to the animals being studied.
- Wild Animal Initiative will continue to evaluate the costs and benefits of biotelemetry. We hope to identify opportunities to collect highly detailed individual-level data without burdening the animals involved.
Introduction
Biotelemetry devices (“biologgers”) can monitor behavioral and physiological indicators of welfare status in real time, and couple these data with precise information about environmental context (Brown et al. 2013). Biologgers consist of a suite of sensors attached to an animal. The different sensors typically record location, motion, or other biological and environmental features multiple times a day (Cooke et al. 2004). The data collection is remote, meaning that the human observer does not operate in the field except to capture the animals and attach the devices.
In one such study, ornithologists outfitted a female white-tailed sea eagle (Haliaeetus albicilla) with a biologger that recorded her movement, circadian rhythm, behavioral profile, and body temperature (Krone et al. 2009). In this article, I use this case study to illustrate biotelemetry’s potential to offer insights on wild animals’ welfare.
The biologger in this case comprised two radio transmitters, a battery pack, and sensors monitoring location, temperature, vertical motion, and horizontal motion. (Figure 1). At 170 g, the device weighed 3.5% as much as the eagle. The study ended after only five months when the eagle unexpectedly died.

B.

Figure 1
A) The biologger attached to the white-tailed sea eagle (Haliaeetus albicilla). Adapted from Krone et al. (2009). B) An eagle outfitted with a biologger comparable to the “backpack” style used in the sea eagle case study. Photo credit: Alan Schumacher/USDA Wildlife Services.
As with many biotelemetry studies, this one focused on a rare species (the white-tailed sea eagle) and collected data to inform its conservation. Although welfare was not the focus of the project, it nonetheless proved data highly relevant to individual welfare because it documented the eagle’s dying process in unprecedented detail. This illustrates how biologgers could be used to understand the welfare burdens of different causes of death.
Welfare-relevant conclusions from biotelemetry
Cause of death
GPS data enabled the researchers to identify both the immediate cause of death and the broader environmental context for it.
Because the biologger transmitted location data every 5 minutes, the researchers were able to determine the precise time and location of the eagle’s death and to recover her corpse as soon as possible — overcoming major challenges in cause-of-death research. Through necropsy, they determined she died of lead poisoning (Krone et al. 2009).
Because the data stream was continuous for 5 months, there was strong evidence that the eagle had ingested lead while foraging within her territory. Even though the eagle had a high-quality habitat that was protected in a conservation reserve, she was still at risk of lead exposure because of legal hunting in her environment (Krone et al. 2009).
Welfare while dying
The biologger fitted to this eagle also included accelerometers, which provided a high-resolution behavioral profile. Activity readings were taken 8 times per second for the entire duration of the study. This frequency enabled researchers to characterize the eagle’s usual circadian rhythm, as well as the start of her sickness period and her dying period. The sickness and dying periods each lasted 11 days (Krone et al. 2009).
With an understanding of the animal’s typical daily rhythms, it was clear when she started showing abnormal behaviors that indicated disease: changes to the timing of flight and rest and increases in the frequency of rest periods (Veissier et al. 1989, Scheibe et al. 1999). The eagle eventually ceased all activity. This extreme lethargy signalled the beginning of her dying process (Krone et al. 2009). Her body temperature also dropped a total of 15℃, coinciding with the decreases in activity (Krone et al. 2009). This physiological and behavioral profile provides a basis for inferring welfare status during the dying process (Figure 2).

Figure 2
Using biotelemetry data to estimate welfare. The activity and body temperature timelines approximate the actual data Krone et al. (2009) collected from a biologger attached to a white-tailed sea eagle (Haliaeetus albicilla) in the weeks preceding her death. The inferred feelings timeline estimates the subjective mental states the eagle may have been experiencing at the same time.
I compared the eagle’s behavioural and physiological profiles over time to her baseline readings in order to estimate her mental state (Beausoleil et al. 2018). Although it may be hard to ever know with certainty how physiological conditions relate to subjective feelings in other species, we can make reasonable guesses given similarities between our own and other animals’ needs.
The eagle’s lethargy could be associated with negative feelings of malaise. The sickness and dying phases spanned 22 days, so the eagle would have also had increasing feelings of hunger, as she was not eating during this time (Mellor and Beausoliel 2015). The necropsy showed total depletion of fat reserves (Krone et al. 2009). Since eagles eagles ideally eat about 5% of their body weight daily to maintain peak body condition, (Fevold and Craighead 1958), it is reasonable to assume that this loss of reserves and lack of hunting activity would lead to feelings of severe hunger and discomfort. Lastly, the 15º C drop in body temperature would likely lead to extreme discomfort associated with coldness (Mellor and Beausoliel 2015).
Advantages of biologgers for wild animal welfare studies
Recovering corpses
For studying death in the wild, the traditional methods such as short-duration video or corpse-recovery surveys have well-known limitations. Corpses are encountered by chance (Dell et al. 2014). Most are not recovered because they are decomposed or scavenged, making it incredibly difficult to estimate the relative frequencies of different causes of death in a population (Tavecchia et al. 2011).
Biotelemetry has major advantages for cause-of-death research because it provides detailed information on when and where animals die (Klaassen et al. 2014). As a result, most of the deaths can be attributed to a specific cause (Figure 3). The positional data can also help researchers rapidly and systematically recover corpses (Calvete et al. 2002).

Figure 3
Causes of death in eagles who died during biotelemetry studies. Data from a cause-specific mortality database (Hill et al. 2019a).
Knowing the prevalence and severity of different causes of death is important, because the amount of suffering that an animal experiences while dying is largely determined by the cause and manner of death (Beausoleil et al. 2016). Vehicle collisions, hunting with firearms, or electrocution may cause near-instantaneous death, whereas disease, starvation, or intoxication may weaken the animal over an extended period (22 days in our eagle case study).
Beyond simply identifying the cause of death, comparative studies could allow us to infer the experiences of animals that die from different causes, and how the prevalence of these causes varies by life stage or environment. Such knowledge would allow wild animal welfare advocates to prioritize preventing the most painful manners of death.
Observing behavior
Currently, most welfare assessments are done by in-person human observation (Bailey et al. 2018). But biotelemetry allows continuous data collection from almost any location an animal may occupy — even where people cannot follow (Ropert-Coudert and Wilson 2005).
The ability to record quantitative behavioral profiles anywhere the animal goes allows for much more informed welfare estimates. For example, behavioral profiles can show sickness behaviors or other abnormal trends in function and condition (Brown et al. 2013, Adelman et al. 2014). This multidimensional information allows us to understand an animal’s welfare status in the context of events and environmental variables that matter at the scale of an individual’s preferences and perceptions (Bisson et al. 2009, Martins et al. 2014, McClune et al. 2015, Clemente al. 2016).
Observing community interactions
Because biologgers minimally distort behavior and can transmit data from any location, they are particularly well-suited to observing individuals’ interactions with their communities. For example, animals in different environments could be monitored as they cope with the various habitats that they encounter. This analysis would provide an animal’s-eye view on habitat quality and show to what extent an animal’s welfare might be improved or diminished by altering the environment (Lima and Zollner 1996, Nicol et al. 2020).
By pairing biotelemetry with an understanding of community interactions, it may be possible to show how community structure degrades or enhances the average welfare of each animal community-member. For example, in a community with two species that cooperate beneficially, biotelemetry could monitor the degree to which stress is reduced when animals have the opportunity to interact (Hammers et al. 2019).
Concerns about biotelemetry research
Technological limitations
Cost is the largest barrier to scaling wildlife biotelemetry research. Devices typically range from hundreds to thousands of dollars per unit (Cooke et al. 2004). Because of the high cost, sample sizes of biotelemetry studies are typically insufficient to predict a welfare outcome before it occurs, even though the data itself is reflective of welfare status (Lindberg and Walker 2007, Costantini and Møller 2013, Naef-Daenzer and Grüebler 2014).
Additionally, while biologgers have been shrinking in size due to advances in battery technology, they are still most commonly only usable on larger animals (Cooke et al. 2004). Unless the weight barriers can be overcome, the vast majority of animals — small invertebrates — are impossible to study with biotelemetry.
Among marine invertebrates, biotelemetry is generally only feasible for individuals weighing more than 400 g. If this threshold were decreased to 40 g, then ten times more species could be studied, as could larvae and juveniles (O’Dor 2002). In terrestrial insects, biotelemetry studies have been limited to a few large arthropods, such as locusts or hawkmoths. Insect biologgers usually weigh less than 1 g, but their battery life is 3 hours or less (Sato and Maharbiz 2010). This temporal limitation nearly eliminates the ability to research invertebrate welfare through biologgers.
Welfare harms
Biologgers harm the animals wearing them (Hawkins 2004). Death during the tagging process can occur either at capture, directly after release, or from physical attrition over the course of the study (Casas et al. 2015, Weiser et al. 2016, Lameris et al. 2018). For example, the eagle in our case study was caught with a noose, a particularly stressful and risky method for capturing birds (Kautz and Seamans et al. 1980).
Even in cases with no tag-related mortality (for example, Goldsmith et al. 2017), the devices can have sublethal impacts such as exhaustion, injury, reduced body condition, and the disruption of social activities and pair bonding (Weiser et al. 2016, Brlík et al. 2020, Hamelin and James 2018, Lameris et al. 2018; but see Niles et al. 2010 which found no sublethal impacts).
The eagle in our case study appeared to have no negative symptoms from the biologger (Krone et al. 2009). However, the authors noted several instances where the bird was at rest during abnormal periods of the day. The authors theorized that stresses such as hunters, tourists, inclement weather, or even sub-lethal lead exposures, may have disrupted the eagle (Krone et al. 2009). But back-mounted biologgers such as the one worn by this eagle (Figure 4) have been documented to cause significant drag that impairs flight (Irvine et al. 2007, Meyer et al. 2015). Therefore, the device itself could have periodically exhausted the bird and necessitated abnormal rest periods.
Conclusions
Biotelemetry’s scientific applications are promising for wild animal welfare research. For example, biologgers can be used to understand the prevalence of different causes of death. Biologgers provide behavioral and physiological profiles at such an extraordinary level of detail that they can potentially be used to infer animals’ mental states. As this study illustrates, these details can be discovered not only throughout an animal’s life, but uniquely, during their sickness and dying process, potentially revealing important information about the harms associated with different causes of death. Finally, by linking physiological and behavioral data with precise location data, biotelemetry gives unprecedented insight into the links between animals and their environment in real time.
The shift from direct human observation to remote monitoring is not without costs to the animals themselves. Biologgers’ weight and drag is substantial, and there are many documented instances of welfare harms and tag-related deaths. Therefore, it remains unclear whether biologgers in their current forms are justifiable or necessary for conducting wild animal welfare research. We plan to conduct further work to identify the costs and benefits associated with biologgers, best practices for determining when such work is necessary (if ever), and what can be done (if anything) to eliminate welfare harms during remote monitoring studies.
How wild animals die: what we know so far
In part three of this series, Luke Hecht gives an overview of what research in this field has taught us so far about how wild animals die and highlights gaps that seem especially important for welfare biology.
This is the final post in three-part series. Read part one → Read part two →
Key takeaways
- Many studies have investigated the causes of death of adult mammals and birds, but there is a lack of data on the deaths of juvenile animals, as well as fish and insects.
- Large, adult animals are more likely to be killed by humans than by any other cause. Small-bodied animals and juveniles are more susceptible to predation by other wild animals.
- Technological improvements in underwater monitoring will improve our understanding of wild fish mortality.
- Insects often die in very different ways from larger animals. Most of what we know about insects’ deaths comes from agricultural pest control research.
- Populations need to be studied under a range of conditions to predict how their cause-specific mortality rates might change under different scenarios.
Introduction
Previously, I wrote about approaches to studying wild animals’ causes of death, with the goal of making work in this field maximally useful for understanding wild animal welfare. Cause of death has not received sufficient research attention relative to its significance to wild animal welfare. The overwhelming majority of the research that does exist is focused on land-dwelling mammals and birds, and primarily on cases where understanding what animals are dying from is instrumental to preventing the extinction of their species. Here, I will give an overview of what research in this field has taught us so far about how wild animals die and highlight gaps that seem especially important for welfare biology.
Cause of death in wild terrestrial vertebrates
Collins and Kays (2011) conducted the first systematic review of cause-specific mortality rates in mammals, selecting only studies that used small radio trackers to monitor animals and document their deaths in a timely manner. They also limited their analysis to adult animals, due to a serious lack of data on juvenile mortality causes. This review found that, overall, predation was the most common cause of death for small-bodied mammals, while human-caused deaths, including hunting and vehicle collision, were the most common causes of death in larger mammals.
In 2019, Hill et al. expanded on this review with a vast amount of new data, including data from juveniles and non-mammalian vertebrates. They found that natural causes of death (predation, disease, starvation), especially predation, were common among juvenile animals irrespective of their species’ typical adult body size. For mammals and reptiles, predation was roughly twice as frequent in juveniles as in adults, with predation accounting for more than 95% of documented deaths of juvenile reptiles (Figure 1). Birds, on the other hand, exhibited very similar cause-specific mortality rates between juvenile and adult age classes.
The authors note that their dataset probably still greatly underestimates natural causes of death, considering that these deaths are often harder to detect. Although smaller animals exhibit the highest natural mortality rates, they are harder to monitor. Research to date has also focused on populations that come into conflict with or are used by humans. These factors combine to make the most numerous deaths in the wild the least understood.

Figure 1
Cause-specific mortality rates separated by age and taxonomic class. Adapted from Hill et al. (2019).
Hill et al. provide an excellent summary of mortality causes, but many of these categories — especially predation, accident and disease — encompass a huge degree of variation in experience. For example, 18% of New Zealand sea lions die from tuberculosis, while 24% die as a result of violence from other sea lions (Lenting et al. 2019). Similarly, approximately half of American black bears die as cubs, often as a result of attacks by older male bears (LeCount 1987). In their study area of northern Pennsylvania, Alt (1984) estimated that 5% of cubs drowned in their winter dens as a result of flash flooding. This was 3-5 times more likely to occur when dens were made in root cavities or excavated soil. Juvenile bears also frequently die from disease (e.g. Chomel et al. 1998). For example, a grizzly cub suffering from canine hepatitis died live on camera in Katmai National Park beside their bewildered mother and siblings. Welfare interventions will need to account for all this variation in individual wild animals’ experiences of death.
Much of the data on birds’ deaths comes from urban wildlife hospitals, where traumatic injury is often the apparent leading cause of death among adult birds, while disease and malnutrition is responsible for the majority of juvenile deaths (e.g. Stenkat et al. 2013). However, these statistics are certain to be biased by the kinds of birds and causes of death that people are most likely to come into contact with. For example, although many traumatic injuries are attributable to domestic cats, birds attacked by predatory raptors are rarely admitted (c.f. Palma et al. 2006). Studies of radio-tagged wild birds report predation and hunting as the leading causes of death (Hill et al. 2019). Some disease mortality can also be linked to hunting practices, as the importation of birds to stock hunting grounds can contribute to outbreaks (Buenestado 2009). Migratory birds present a special challenge for researchers, since many of their deaths occur in transit over long distances, where it may be impossible to recover their corpses. For example, out of 51 probable deaths among satellite-tracked migratory raptors, Klaasen et al. (2014) were only able to confirm 10, among which the leading causes of death were collision with man-made structures and exhaustion during migration.
The progress made in the last decade of cause-specific mortality research is encouraging, but there is much more to be learned, especially about more numerous, smaller-bodied animals. Little is known about the causes of death among prairie dogs, for instance, except that predation is common and plague occasionally wipes out entire colonies (Crosby and Graham 1986; Stapp et al. 2004). Studies of wild reptiles, especially snakes and turtles, consistently indicate road traffic accidents as a leading cause of death (e.g. Himes et al. 2002), although most juveniles are killed by predators (e.g. Butler and Sowell 1996). These gaps in our knowledge of wild animal mortality seem likely to be filled as radio tracking devices are made smaller, lighter and more resilient over the next decade (Kays et al. 2015).
Cause of death in wild fish
Among vertebrates, the most neglected group in the current body of cause-specific mortality research is wild fish. Of the papers that do touch on causes of death in wild fish, many are case reports focusing on the risks of disease outbreaks to human health or the fishing industry. Case reports can be valuable for demonstrating the presence of specific risks that some number of fish do die from (Krkošek 2017). For example, Sterud et al. (2007) detected an outbreak of the parasite Tetracapsuloides bryosalmonae in an Atlantic salmon population that appeared to be their most common cause of death during the study period. This parasite is probably in constant circulation among the fish, and outbreaks have been documented in many other populations, but it is impossible to say whether these results are a representative snapshot of the parasite’s effect on mortality or what alternative causes of death occur in the population.
Most effective studies of terrestrial animals’ cause of death rely on recovering the corpses of tagged individuals, as discussed in my previous post. However, this is clearly more challenging to do with fish. Radio signals for tracking individuals are relatively ineffective underwater, and corpses are much less likely to be recovered (c.f. Benelli and Pozzebon 2013). Several studies have cleverly taken advantage of avian predators naturally retrieving radio-tagged fish from the water and depositing their eaten corpses on shore. For example, Koed et al. (2006) estimated that 39% and 12% of juvenile salmon and brown trout, respectively, were preyed upon by cormorants in the estuary where their study was set. Approximately a further 10% of salmon and trout were killed by cormorants in the adjoining river, while 3-6% were preyed upon by pike, a predatory fish. A similar study by Dieperink et al. (2001) recorded that 65% of juvenile trout were eaten by cormorants and herons while migrating downstream to the Baltic Sea. Fritts and Pearsons (2004) used another predator-based approach to quantify predation, analyzing the stomach contents of smallmouth bass to estimate their annual consumption of salmon. Taken at face value, their results suggested that smallmouth bass accounted for only around 4% of salmon mortality in this system, though the authors noted that this was probably an underestimate. Despite the special challenges involved in recovering the corpses of wild fish, it is at least possible to study the impact of specific predators on fish populations.
Technological progress is proving especially important for studying cause of death in the marine environment. In one recent study, fish were implanted with acoustic transmitters that enabled the researchers to detect predation events occurring within range of their underwater receiver array (Weinz et al. 2020). They inferred that approximately one third of tagged fish were preyed upon over the four months following their release. Several factors could be confounding this result, in both directions. For one, the invasive procedure required to implant the fish with acoustic transmitters could have made these particular fish more susceptible to predation than average. This would be consistent with other studies showing that fish caught and released are more vulnerable due to physical injuries or stress (e.g. Raby et al. 2013). On the other hand, some predation events were likely missed because they occurred out of range of the acoustic array. As the range of underwater tracking improves and the size of transmitting devices decreases so as to cause less harm to the tagged individuals, we should expect to see much more data on the lives and deaths of fish and other marine animals.
Cause of death in insects
Insects are among the most numerous and diverse animals, but also the smallest. Their diversity and size makes them especially difficult to monitor on an individual level. At the same time, some insect species are viewed as pests, and so there is commercial interest in understanding their natural causes of death to devise more effective management strategies (Roux and Baumgärtner 1998). This has motivated a handful of studies reviewing the causes of death of agriculturally relevant insects (Table 1).
Some of these studies use a combination of field observations and population modeling to estimate by how much the population’s overall mortality rate would be reduced if a given cause of death could be eliminated. For example, if predators disproportionately target weak or sick prey, then even if many individuals ultimately die from predation, eliminating their predators may have only a small effect on the overall mortality rate because the same individuals who are vulnerable to predation are also vulnerable to dying soon from disease or starvation.

Table 1
Summarized results from eight studies on cause-specific mortality in six insect species from a pest management context.
Determining the significance of competing mortality risks
Future interventions seeking to improve wild animal welfare should account for how actions to protect animals from specific causes of death could influence population sizes, lifespans, and alternative causes of death. Compensatory mortality, where mortality due to a certain cause is replaced by mortality due to another cause when the first is removed, has been noted in many diverse species and ecosystems. For example, Hostetter et al. (2012) reported that cormorants along the Columbia River in Oregon selectively predate poorer-condition juvenile steelhead salmon en route to the sea. Had they not been killed by cormorants, these same individuals would likely have been among the first to succumb to harsh conditions and competition during their first winter in the ocean (Hurst 2007). Similarly, in a classic study on mule deer, Bartmann et al. (1992) found that removal of coyotes over winter reduced the rate of mule deer deaths by predation, but did not increase their overall survival rates, as the researchers observed increased mortality due to starvation. Diseased mule deer are also selectively preyed upon by mountain lions (e.g. Krumm et al. 2009), which suggests that some of this predator-caused mortality is compensatory.
On the other hand, some cause-specific mortality really is irreplaceable. Achhami et al. (2020) demonstrated this for plant chemical defenses against wheat stem sawflies, while Cooley et al. (2009) showed that other causes of death are not reduced in populations of mountain lions hunted by humans. Bergman et al. (2015) proposed that the degree to which mortality due to a particular cause is compensatory depends on ecological context. For example, if chronic illness made animals less effective at competing for food in a dense population, death by starvation may occur first and compensate for some disease-related mortality in this hypothetical population. If food later became more accessible, we might observe an apparent increase in mortality caused by diseases that are now able to run their course. In addition to studying mortality dynamics in a snapshot of a population’s present conditions, research should model how mortality risks compete under different conditions (Siler 1979) (Figure 2).

Figure 2
Three hypothetical scenarios for the dynamics of mortality due to predation on a prey population. In all scenarios, baseline mortality in the absence of predators is 50 individuals per year. If predator-induced mortality is completely additive (purple diamonds), 10 more animals dying to predation increases total mortality by 10. If predation is completely compensatory (blue squares), total mortality stays constant despite an increased number of deaths by predation; instead, there are commensurately fewer deaths by other causes. In most cases, mortality due to a given cause is likely to be partially compensatory (green circles), lying somewhere between these two extremes. In this model, low rates of predation are mostly compensatory, perhaps removing old or sick individuals from the population. As the intensity of predation increases, a larger proportion of healthy animals are killed and so predation mortality becomes increasingly additive.
Conclusions
A relatively comprehensive snapshot of cause of death in the wild is emerging for terrestrial mammals, birds, and reptiles, thanks to mainstream research in ecology and conservation (Hill et al. 2019). For example, we can see that predation is the leading natural cause of death, especially in juveniles (Figure 1). However, this account is still likely to be heavily biased, since most studies focus on adults and large-bodied species. The focus on large animals could be inflating the number of deaths caused by hunting rather than vehicle collisions, for instance, given that accidents involving small animals often go unreported (Sáenz-de-Santa-María and Tellería 2015). More research on juveniles and small mammals is needed (Table 2). We are also at the stage of considering interventions to improve the welfare of some wild mammals and birds, ranging from pigeon fertility control to reducing feral cat predation. To make these interventions as effective as possible, it would be valuable to understand the replaceability of different sources of mortality.

Table 2
Research gaps and proposed priorities by taxon.
Fish and juvenile amphibians are still seriously lacking in data on cause-specific mortality, especially relating to juveniles. Research on both of these groups is challenged by the fact that they spend some or all of their lives underwater, but biologging technologies — including pop-up satellite archival tags (PSATs) (Tolentino et al. 2017) and acoustic telemetry (Weinz et al. 2020) — could enable more research on cause of death in aquatic environments, especially related to predation.
A surprising amount is known about cause-specific mortality in insects regarded as agricultural pests (Table 1). However, very little research has been done on the deaths of insects with less agricultural relevance. From the existing research, it is clear that insects face challenges that would surprise us. For example, Asiimwe et al. (2006) found that many whiteflies died as a result of poorly understood developmental abnormalities, such as failure to complete metamorphosis. Drowning during rainy weather was one of the foremost causes of death among the moths of Pereira et al. (2007), and plant chemical defenses were responsible for a majority of the deaths of wheat stem sawflies monitored by Acchami et al. (2020).
As many challenges as we know that animals face in the wild, some may yet be masked by the urgent threats they face from humans through hunting, fishing, extermination, and competition for resources. Humans should leave more space for wildlife, and try not to make their existence harder than it already is, but we should also be willing to go further. Learning more about cause-specific mortality in different populations and environmental contexts can inform plans for the most effective actions to improve wild animal welfare.
References
Achhami, Buddhi B., Robert K. D. Peterson, Jamie D. Sherman, Gadi V. P. Reddy, and David K. Weaver. 2020. ‘Multiple Decrement Life Tables of Cephus Cinctus Norton (Hymenoptera: Cephidae) across a Set of Barley Cultivars: The Importance of Plant Defense versus Cannibalism’. PLOS ONE 15 (9): e0238527. https://doi.org/10.1371/journal.pone.0238527.
Andis, M. D., and C. L. Meek. 1985. ‘Mortality and Survival Patterns for the Immature Stages of Psorophora Columbiae’. Journal of the American Mosquito Control Association 1 (3): 357–62.
Asiimwe, P., J. S. Ecaat, M. Otim, D. Gerling, S. Kyamanywa, and J. P. Legg. 2007. ‘Life-Table Analysis of Mortality Factors Affecting Populations of Bemisia Tabaci on Cassava in Uganda’. Entomologia Experimentalis et Applicata 122 (1): 37–44. https://doi.org/10.1111/j.1570-7458.2006.00487.x.
Bartmann, Richard M., Gary C. White, and Len H. Carpenter. 1992. ‘Compensatory Mortality in a Colorado Mule Deer Population’. Wildlife Monographs, no. 121: 3–39.
Benelli, G., Pozzebon, A., & Reaz, M. B. I. 2013. ‘RFID under water: Technical issues and applications’ (pp. 379-395). Rijeka: InTech. https://doi.org/10.5772/53934.
Bergman, Eric J., Paul F. Doherty, Gary C. White, and A. Andrew Holland. 2015. ‘Density Dependence in Mule Deer: A Review of Evidence’. Wildlife Biology 21 (1): 18–29. https://doi.org/10.2981/wlb.00012.
Buenestado, F. J., Ferreras, P., BLANCO‐AGUIAR, J. A., Tortosa, F. S., & Villafuerte, R. 2009. ‘Survival and causes of mortality among wild Red‐legged Partridges Alectoris rufa in southern Spain: implications for conservation’. Ibis 151 (4): 720-730. https://doi.org/10.1111/j.1474-919X.2009.00952.x.
Butler, J. A., & Sowell, S. 1996. ‘Survivorship and predation of hatchling and yearling gopher tortoises, Gopherus polyphemus’. Journal of Herpetology 30 (3): 455-458. https://doi.org/10.2307/1565195.
Chomel, B.B., R.W. Kasten, G. Chappuis, M. Soulier, and Y. Kikuchi. 1998. ‘Serological Survey of Selected Canine Viral Pathogens and Zoonoses in Grizzly Bears (Ursus Arctos Horribilis) and Black Bears (Ursus Americanus) from Alaska: -EN- -FR- -ES-’. Revue Scientifique et Technique de l’OIE 17 (3): 756–66. https://doi.org/10.20506/rst.17.3.1134.
Collins, C., and R. Kays. 2011. ‘Causes of Mortality in North American Populations of Large and Medium-Sized Mammals’. Animal Conservation 14 (5): 474–83. https://doi.org/10.1111/j.1469-1795.2011.00458.x.
Cooley, Hilary S., Robert B. Wielgus, Gary M. Koehler, Hugh S. Robinson, and Benjamin T. Maletzke. 2009. ‘Does Hunting Regulate Cougar Populations? A Test of the Compensatory Mortality Hypothesis’. Ecology 90 (10): 2913–21. https://doi.org/10.1890/08-1805.1.
Crosby, Lyle A., and Randy Graham. 1986. ‘Population Dynamics and Expansion Rates of Black-Tailed Prairie Dogs’. Proceedings of the Vertebrate Pest Conference 12 (12). https://escholarship.org/uc/item/23t218h1.
Dieperink, C., S. Pedersen, and M. I. Pedersen. 2001. ‘Estuarine Predation on Radiotagged Wild and Domesticated Sea Trout (Salmo Trutta L.) Smolts’. Ecology of Freshwater Fish 10 (3): 177–83. https://doi.org/10.1034/j.1600-0633.2001.100307.x.
Fritts, Anthony L., and Todd N. Pearsons. 2004. ‘Smallmouth Bass Predation on Hatchery and Wild Salmonids in the Yakima River, Washington’. Transactions of the American Fisheries Society 133 (4): 880–95. https://doi.org/10.1577/T03-003.1.
Hill, Jacob E., Travis L. DeVault, and Jerrold L. Belant. 2019. ‘Cause-Specific Mortality of the World’s Terrestrial Vertebrates’. Global Ecology and Biogeography 28 (5): 680–89. https://doi.org/10.1111/geb.12881.
Himes, J. G., Hardy, L. M., Rudolph, D. C., & Burgdorf, S. J. 2002. ‘Growth rates and mortality of the Louisiana pine snake (Pituophis ruthveni)’. Journal of Herpetology 36 (4): 683-687. https://doi.org/10.2307/1565941.
Hostetter, Nathan J., Allen F. Evans, Daniel D. Roby, and Ken Collis. 2012. ‘Susceptibility of Juvenile Steelhead to Avian Predation: The Influence of Individual Fish Characteristics and River Conditions’. Transactions of the American Fisheries Society 141 (6): 1586–99. https://doi.org/10.1080/00028487.2012.716011.
Hurst, T. P. 2007. ‘Causes and Consequences of Winter Mortality in Fishes’. Journal of Fish Biology 71 (2): 315–45. https://doi.org/10.1111/j.1095-8649.2007.01596.x.
Jones, Davy. 1982. ‘Predators and Parasites of Temporary Row Crop Pests: Agents of Irreplaceable Mortality or Scavengers Acting Prior to Other Mortality Factors?’ Entomophaga 27 (3): 245–65. https://doi.org/10.1007/BF02374809.
Karut, K., and S. E. Naranjo. 2009. ‘Mortality Factors Affecting Bemisia Tabaci Populations on Cotton in Turkey’. Journal of Applied Entomology 133 (5): 367–74. https://doi.org/10.1111/j.1439-0418.2008.01369.x.
Kays, Roland, Margaret C. Crofoot, Walter Jetz, and Martin Wikelski. 2015. ‘Terrestrial Animal Tracking as an Eye on Life and Planet’. Science 348 (6240). https://doi.org/10.1126/science.aaa2478.
Klaassen, R. H., Hake, M., Strandberg, R., Koks, B. J., Trierweiler, C., Exo, K. M., ... & Alerstam, T. 2014. ‘When and where does mortality occur in migratory birds? Direct evidence from long‐term satellite tracking of raptors’. Journal of Animal Ecology 83 (1): 176-184. https://doi.org/10.1111/1365-2656.12135.
Koed, Anders, Henrik Baktoft, and Brian Daniel Bak. 2006. ‘Causes of Mortality of Atlantic Salmon (Salmo Salar) and Brown Trout (Salmo Trutta) Smolts in a Restored River and Its Estuary’. River Research and Applications 22 (1): 69–78. https://doi.org/10.1002/rra.894.
Krkošek, Martin. 2017. ‘Population Biology of Infectious Diseases Shared by Wild and Farmed Fish1’. Canadian Journal of Fisheries and Aquatic Sciences, January. https://doi.org/10.1139/cjfas-2016-0379.
Krumm, Caroline E., Mary M. Conner, N. Thompson Hobbs, Don O. Hunter, and Michael W. Miller. 2010. ‘Mountain Lions Prey Selectively on Prion-Infected Mule Deer’. Biology Letters 6 (2): 209–11. https://doi.org/10.1098/rsbl.2009.0742.
LeCount, Albert L. 1987. ‘Causes of Black Bear Cub Mortality’. Bears: Their Biology and Management 7: 75–82. https://doi.org/10.2307/3872610.
Lenting, B., B. Gartrell, A. Kokosinska, P. J. Duignan, S. Michael, S. Hunter, and W. D. Roe. 2019. ‘Causes of Adult Mortality in Two Populations of New Zealand Sea Lions (Phocarctos Hookeri)’. Veterinary and Animal Science 7 (June): 100057. https://doi.org/10.1016/j.vas.2019.100057.
Naranjo, Steven E., and Peter C. Ellsworth. 2005. ‘Mortality Dynamics and Population Regulation in Bemisia Tabaci’. Entomologia Experimentalis et Applicata 116 (2): 93–108. https://doi.org/10.1111/j.1570-7458.2005.00297.x.
Palma, L., Beja, P., Pais, M., & Cancela Da Fonseca, L. 2006. ‘Why do raptors take domestic prey? The case of Bonelli's eagles and pigeons’. Journal of Applied Ecology 43 (6): 1075-1086. https://doi.org/10.1111/j.1365-2664.2006.01213.x.
Pereira, E. J. G., M. C. Picanço, L. Bacci, A. L. B. Crespo, and R. N. C. Guedes. 2007. ‘Seasonal Mortality Factors of the Coffee Leafminer, Leucoptera Coffeella’. Bulletin of Entomological Research 97 (4): 421–32. https://doi.org/10.1017/S0007485307005202.
Peterson, Robert K. D., Ryan S. Davis, Leon G. Higley, and Odair A. Fernandes. 2009. ‘Mortality Risk in Insects’. Environmental Entomology 38 (1): 2–10. https://doi.org/10.1603/022.038.0102.
Raby, Graham D., Jessica R. Packer, Andy J. Danylchuk, and Steven J. Cooke. 2014. ‘The Understudied and Underappreciated Role of Predation in the Mortality of Fish Released from Fishing Gears’. Fish and Fisheries 15 (3): 489–505. https://doi.org/10.1111/faf.12033.
Roux, Olivier, and Johann Baumgärtner. 1998. ‘Evaluation of Mortality Factors and Risk Analysis for the Design of an Integrated Pest Management System’. Ecological Modelling 109 (1): 61–75. https://doi.org/10.1016/S0304-3800(98)00035-0.
Sáenz-de-Santa-María, A., & Tellería, J. L. 2015. ‘Wildlife-vehicle collisions in Spain’. European Journal of Wildlife Research 61 (3): 399-406. https://doi.org/10.1007/s10344-015-0907-7.
Siler, W. 1979. ‘A competing‐risk model for animal mortality’. Ecology 60 (4): 750-757. https://doi.org/10.2307/1936612.
Stapp, Paul, Michael F. Antolin, and Mark Ball. 2004. ‘Patterns of Extinction in Prairie Dog Metapopulations: Plague Outbreaks Follow El Ninì Events’. Frontiers in Ecology and the Environment 2 (5): 235–40. https://doi.org/10.1890/1540-9295(2004)002[0235:POEIPD]2.0.CO;2.
Stenkat, J., Krautwald-Junghanns, M. E., & Schmidt, V. 2013. ‘Causes of morbidity and mortality in free-living birds in an urban environment in Germany’. Ecohealth 10 (4): 352-365. https://doi.org/10.1007/s10393-013-0868-9.
Sterud, E, T Forseth, O Ugedal, Tt Poppe, A Jørgensen, T Bruheim, H Fjeldstad, and Ta Mo. 2007. ‘Severe Mortality in Wild Atlantic Salmon Salmo Salar Due to Proliferative Kidney Disease (PKD) Caused by Tetracapsuloides Bryosalmonae (Myxozoa)’. Diseases of Aquatic Organisms 77 (October): 191–98. https://doi.org/10.3354/dao01846.
Tolentino, Emily R., Russell P. Howey, Lucy A. Howey, Lance K. B. Jordan, R. Dean Grubbs, Annabelle Brooks, Sean Williams, Edward J. Brooks, and Oliver N. Shipley. 2017. ‘Was My Science Project Eaten? A Novel Approach to Validate Consumption of Marine Biologging Instruments’. Animal Biotelemetry 5 (1): 3. https://doi.org/10.1186/s40317-016-0117-4.
Weinz, Amy A., Jordan K. Matley, Natalie V. Klinard, Aaron T. Fisk, and Scott F. Colborne. 2020a. ‘Identification of Predation Events in Wild Fish Using Novel Acoustic Transmitters’. Animal Biotelemetry 8 (1): 28. https://doi.org/10.1186/s40317-020-00215-x.
Methods for studying wild animals’ causes of death
In part two of this series, Luke Hecht introduces some of the tools and approaches used to study wild animals’ causes of death.
Key takeaways
- Cause of death is an important area of wild animal welfare research.
- Accurate cause-specific mortality rates are difficult to obtain.
- Multistate modeling can provide insight where information about cause-specific mortality rates is incomplete.
- Experimental studies and improved monitoring technology can improve our ability to diagnose cause of death, estimate cause-specific mortality rates, and understand compensatory mortality.
- Future research should identify and focus on the causes of death that involve the most suffering.
In a world with perfect monitoring of wild populations, biologists could be immediately alerted to the death of an animal, show up on the scene, and perform a necropsy to determine the animal’s precise cause of death. They would also know everything about the age and health history of this animal, having monitored them from birth. Unfortunately, it is not practical to monitor most wild populations at nearly this level of detail. Our understanding of how and why wild animals die must be pieced together from statistical models and sparse empirical data. In this post, I will introduce some of the tools and approaches used to study wild animals’ causes of death.
Death assemblages
The most straightforward way to study wild animals’ causes of death is to scour a habitat for corpses. The resulting collection is sometimes called a “death assemblage.” When the body of a recently deceased animal is recovered, indicators such as injuries, signs of disease, and stomach contents may make it possible to determine what killed them (Behrensmeyer and Miller 2012).
Determining cause of death from serendipitously recovered corpses depends on finding animals soon after their deaths. Death assemblage surveys are therefore most useful for animals whose remains are more likely to be discovered, like coastal marine mammals. Their large size means they take a while to decay, and their bodies often wash up on beaches where they are highly visible and quickly reported to authorities or researchers (Tinker et al. 2016). Recovery of well-preserved corpses is much less common for animals who are small or live in lush environments like forests, which typically provide more concealment and support more rapid decomposition.
The probability of recovering a corpse can also be biased by whatever caused the animal’s death (Gerber et al. 2004). For example, the bodies of animals who were killed by predators may be less likely to be recovered because they are almost entirely consumed on the spot or are carried away from the site (e.g. hyenas stashing bones of prey, Kuhn 2005). On the other hand, animals who die from starvation, disease, old age, or something else that leads to their gradual weakening may attempt to conceal themselves in their final hours. While their bodies are likely to remain intact for longer, they may go unnoticed by researchers.
Certain causes of death are also inherently more difficult to diagnose, so researchers need to be wary of simply ignoring data in an “unknown” category that may be disproportionately filled with instances of hard-to-distinguish causes of death. For example, in a survey of bones found in a hyena den, presumably belonging to their past prey, Kuhn (2005) identified roughly a quarter of the specimens to species level. Without further information, any quantitative claims about the composition of the local ecosystem based on these data would have to be taken with serious caution because the remaining 75% of “unknown” specimens might be drawn unequally from underlying populations. For example, animals who are morphologically similar to other species, or animals who are not preferred prey for hyenas, might be underrepresented in resulting estimates.
Bias is a pervasive problem in cause of death surveys. A naive survey of rabbit corpses in a particular forest might conclude that most recent deaths were attributable to some rapidly debilitating disease, while in fact the majority have been carried off by birds of prey without a trace. To estimate the true rates of alternative causes of death from corpse recovery data, researchers need to account for the fact that the forensic clues to certain causes of death may be more or less detectable than others, depending on a host of circumstances.
Accounting for incomplete information
The inevitable incompleteness of corpse recovery surveys can be partially offset by explicitly modeling sources of uncertainty along the chain of events from death by a specific cause, to corpse recovery, to cause identification. This approach is sometimes called multistate modeling (Hougaard 1999; Gauthier and Lebreton 2009). These models make a distinction between the objective chain of events that occurred to an individual animal and the observable evidence of those events (Koons et al. 2014). For example, researchers generally do not directly observe rodents being preyed on by raptors. A fraction of predation events leave behind bits of fur or the partially eaten body of the rodent. Researchers then have a particular probability of encountering these bits of evidence and deducing the correct cause of death.
Box 1
To illustrate what a multistate model looks like in practice, imagine that a flock of birds begins to roost every day in a tree outside where you live. The foliage is dense, so you cannot see all of the roosting birds at any one time through your window. One of the birds (“Bird X”) has distinctive markings, with which you can usually distinguish them from the others. One day, you can’t see Bird X in the tree. Your first worry is that Bird X might have died, but then you remember that they might just be out of view behind a branch. A week passes and Bird X still hasn’t been seen in the tree. Is it more likely that you simply have not caught sight of Bird X for seven days in a row, or that Bird X died sometime in the week?
This problem can be solved by estimating the exact proportion of birds in the tree who can be seen on any given day (“detection probability”), the probability of recognizing the identity of Bird X if you set eyes on them (“identification probability”), and the average daily survival probability for each bird in this population. These can then be incorporated into a model where Bird X is considered to be in one of two states: alive or dead. Using the model, we can determine whether our series of observations of the tree outside is better explained by Bird X being alive or by being dead. Marescot et al. (2015) applied a similar sort of model to observations of tagged black-tailed deer to estimate their age-specific survival rates and the impact of predation on their population growth.
While multistate modeling is frequently used to estimate survival rates in wild animal populations, similarly nuanced studies of cause of death are rare. This may reflect both a general disinterest in cause-of-death research and a greater confidence in the raw data that does exist. Studying human-caused deaths or species for which there is a good infrastructure for monitoring removes some uncertainty and lessens the need for multistate models. These statistical methods are most important to apply when studying populations where the vast majority of individuals’ deaths go undocumented, leaving room for large biases in the data. For example, Gerber et al. (2004) investigated cause-specific mortality of sea otters. To do so, they took advantage of an extensive network of marine mammal research groups to recover and analyze carcasses that washed up on the beach. Using a demographic model, they estimated that their sample represented just under half of all mortality during the study period. To sample half of all deceased individuals in a wild population of marine mammals is a remarkable achievement, but it highlights how much potential there is for unequal cause-specific recovery rates to produce biased results, especially in less intensively monitored populations. In marine mammals, for example, different predator species may hunt at different distances from shore, or near rocky cliffs as opposed to smooth beaches. Either of those factors could affect the probability of a carcass washing up onshore and being recovered by researchers (Joly et al. 2009).
The biggest weakness of purely observational studies of wild animal mortality is that it is difficult to know what data are missing. The simplest way of overcoming this is to tag a set number of animals at the beginning of a study and only count the remains of tagged individuals. This sort of study design is still subject to biased recovery rates, but the magnitude of bias is limited because corpses of untagged individuals are excluded. Schaub and Pradel (2004) used such an approach to estimate the proportion of white storks killed in airborne collisions with power lines in Switzerland (Figure 1a). They found that simply counting corpses overestimated the proportion of juvenile white storks killed by power lines by about 25%. It makes sense that the bodies of birds killed by power line collisions would be more conspicuous than birds who die otherwise, because the ground around power lines tends to be more exposed to allow for maintenance access. Power lines may also serve as natural concentrating points for associated deaths, whereas deaths by other causes may be uniformly distributed across the landscape.
Limiting analyses to tagged animals is effective at preventing overestimation of particular causes of death, but it can also leave researchers with a lot of missing data from individuals whose bodies were not recovered or who lost their tags. Steps can be taken to improve the recovery rate of tagged animals, such as attaching radio trackers to animals alongside conventional tags (Tavecchia et al. 2011; Figure 1b). Records of when a tagged animal was last seen alive can also complement corpse recovery data, because they allow for independent estimation of survival rates and rates of tag loss. Without this information, corpse recovery data alone cannot distinguish between an animal who is still alive and one who died but was never recovered. Fortunately, technical improvements in the precision and durability of radio trackers are making it practical to do this remotely. Even with relatively basic GPS tags, statistical models can distinguish tag loss or technical failure from actual death of the tagged animal with almost perfect accuracy (Sergio et al. 2018). Electronic tags capable of recording animals’ fine-scale movements or vital signs can also provide evidence related to an animal’s cause of death, such as how sudden it was or what posture the animal adopted in their final moments (Cooke 2008; Brown et al. 2013). Advances in the field of animal remote sensing will be helpful for learning about cause-specific mortality at a level of detail that is currently impossible for researchers to observe.

Figure 1
Multistate models treat wild animals’ fates — observed or unobserved — as the product of a series of conditional probabilities reflecting the combination of events over their life. A) A basic model that considers whether an animal is alive or dead, what caused their death, and whether their corpse was recovered (Schaud and Pradel 2004). B) A more complex model that estimates the probabilities of a bird dying from poisoning or electrocution, and ultimately the probability of a given bird being sighted again alive or dead (Tavecchia et al. 2011). In both of these models, the state transition probabilities are estimated based on the history of encounters between researchers and each tagged animal over the course of a study. For example, if researchers observe an individual after not sighting them for multiple field seasons, then that sighting might both increase the estimated survival rate and decrease the estimated detection rate.
Experimental study of cause-specific mortality
Rather than simply observing the fates of wild animals, experimental approaches directly exclude or introduce specific causes of death. The most common form of this approach is to exclude predators with fencing or other deterrents. For example, Smith et al. (2012) excluded all medium-sized mammalian predators from some nesting grounds of the gopher tortoise, but not others, and found that tortoises born in the predator exclusion zones were almost twice as likely to still be alive one year later. Similarly, Conner et al. (2011) found that exclusion fencing reduced the number of cotton rats killed by mammals. However, increased predation by birds and snakes largely made up for the decreased predation by mammals, especially after a forest fire destroyed much of the undergrowth that could otherwise conceal the rats.
Although most experimental research on cause-specific mortality to date has focused on hunting or predation, a similar approach could be used to study the effectiveness of interventions against other common causes of death, including curable diseases or hunger (c.f. Rickett et al. 2013). However, interventions like supplemental feeding, disease prevention and predator exclusion may result in compensatory mortality, where individuals spared from one cause of death are at elevated risk of dying soon after because of another cause. The most effective way of learning about compensatory mortality risk is to use an experimental rather than observational study design, in conjunction with the same best practices for observational studies as described previously (Bergman et al. 2015).
Outlook for welfare-focused cause of death research
From the perspective of wild animal welfare, understanding cause-specific mortality is morally urgent. A few shifts in the current approach to cause-of-death research will help us determine which issues to prioritize to help animals. Most importantly, much more research should be focused on the most common deaths in the wild: deaths of juvenile animals and members of species with large global populations. Because they are so numerous, these individuals’ experiences of dying are especially important to understand if we value all sentient animals’ welfare equally. These animals tend to be more difficult to monitor due to their smaller body size, but studying their mortality is becoming ever easier with new technology. At the current rate of progress, organisms weighing around one gram could be tracked via GPS within a decade or less (Kays et al. 2015).
One major source of uncertainty in wild animal mortality research is the diagnosis of an animal’s cause of death. This is especially true for low-tech studies, where corpses are encountered by chance, rather than sought at a particular location based on radio tracking and a mortality signal. As a result, corpses may be significantly decomposed or scavenged. Even under ideal circumstances, it can be difficult to determine the order in which apparent injuries occurred. In lieu of perfect monitoring, researchers could perform detailed analysis on a subset of the recovered bodies to evaluate the reliability of their field diagnoses of the rest, as Joly et al. (2009) did with sea otter carcasses. Studies could also be dedicated to intensive monitoring of a relatively small number of animals so that when they die the cause can be known with high confidence and researchers can quickly find their corpses to document field-identifiable characteristics and how these change over the coming days or weeks, as well as estimate recovery rates. The field of taphonomy has long filled a similar supportive role for paleobiology (Behrensmeyer and Miller 2012). Upfront investment in this sort of meta-research could enable subsequent studies of wild animal mortality to accomplish more with less (c.f. Loannidis 2018).
Welfare-focused researchers should aim to identify and focus on the causes of death that entail much more suffering than others. This could involve a reframing of cause-specific mortality research where rather than asking how animals of a given species are likely to die, researchers ask who the victims of a particular cause of death are likely to be. Rather than tracking marmots and determining what proportion were preyed on by cougars, for instance, researchers could monitor cougars using cameras or fecal analysis to learn who their prey were (Popanom et al. 2011). This sort of cause-centric approach might also be appropriate in contexts where a single ultimate cause can lead to several different proximate causes of death which vary in their severity. For example, researchers could survey a forest in the aftermath of a fire and try to determine what proportion of fatalities occurred by burning, asphyxiation, subsequent starvation, or other proximate causes related to the fire (Gutiérrez and Javier de Miguel, 2020). Simply verifying that a certain cause of death occurred at least once in a given population may be useful to inform future work. If so, low-cost cause-specific mortality studies could use a form of rarefaction analysis to judge how comprehensively the causes of death present in a population have been sampled, with a focus on enumerating the causes rather than estimating their relative frequencies.
Efforts to improve the health and lifespans of our fellow humans are supported by publicly available data on the most common causes of death. This is part of how governments decide which public health interventions and fields of medical research to allocate funding to. A comparable wealth of data could help us identify which wild animals need the most urgent aid, and what form that aid should take. In the next part of this series, I will give an overview of some of the data that does exist on the causes of wild animal mortality.
References
Behrensmeyer, Anna K., and Joshua H. Miller. 2012. ‘Building Links Between Ecology and Paleontology Using Taphonomic Studies of Recent Vertebrate Communities’. In Paleontology in Ecology and Conservation, edited by Julien Louys, 69–91. Springer Earth System Sciences. Berlin, Heidelberg: Springer. https://doi.org/10.1007/978-3-642-25038-5_5.
Bergman, Eric J., Paul F. Doherty, Gary C. White, and A. Andrew Holland. 2015. ‘Density Dependence in Mule Deer: A Review of Evidence’. Wildlife Biology 21 (1): 18–29. https://doi.org/10.2981/wlb.00012.
Brown, Danielle D., Roland Kays, Martin Wikelski, Rory Wilson, and A. Peter Klimley. 2013. ‘Observing the Unwatchable through Acceleration Logging of Animal Behavior’. Animal Biotelemetry 1 (1): 20. https://doi.org/10.1186/2050-3385-1-20.
Conner, L. Mike, Steven B. Castleberry, and Anna M. Derrick. 2011. ‘Effects of Mesopredators and Prescribed Fire on Hispid Cotton Rat Survival and Cause-Specific Mortality’. The Journal of Wildlife Management 75 (4): 938–44. https://doi.org/10.1002/jwmg.110.
Cooke, Steven J. 2008. ‘Biotelemetry and Biologging in Endangered Species Research and Animal Conservation: Relevance to Regional, National, and IUCN Red List Threat Assessments’. Endangered Species Research 4 (1–2): 165–85. https://doi.org/10.3354/esr00063.
Gauthier, Gilles, and Jean-Dominique Lebreton. 2008. ‘Analysis of Band-Recovery Data in a Multistate Capture-Recapture Framework’. Canadian Journal of Statistics 36 (1): 59–73. https://doi.org/10.1002/cjs.5550360107.
Gerber, Leah R., M. Tim Tinker, Daniel F. Doak, James A. Estes, and David A. Jessup. 2004. ‘Mortality Sensitivity in Life-Stage Simulation Analysis: A Case Study of Southern Sea Otters’. Ecological Applications 14 (5): 1554–65. https://doi.org/10.1890/03-5006.
Gutiérrez, J. and de Miguel, F. J. 2020. Challenges posed by fires to wild animals and how to help: A literature review, Oakland: Animal Ethics, retrieved from https://www.animalethics.org/fire-wild-animals-help.
Hougaard, Philip. 1999. ‘Multi-State Models: A Review’. Lifetime Data Analysis 5 (3): 239–64. https://doi.org/10.1023/A:1009672031531.
Ioannidis, John P. A. 2018. ‘Meta-Research: Why Research on Research Matters’. PLOS Biology 16 (3): e2005468. https://doi.org/10.1371/journal.pbio.2005468.
Joly, Damien O., Dennis M. Heisey, Michael D. Samuel, Christine A. Ribic, Nancy J. Thomas, Scott D. Wright, and Irene E. Wright. 2009. ‘Estimating Cause-Specific Mortality Rates Using Recovered Carcasses’. Journal of Wildlife Diseases 45 (1): 122–27. https://doi.org/10.7589/0090-3558-45.1.122.
Kays, R., M. C. Crofoot, W. Jetz, and M. Wikelski. 2015. ‘Terrestrial Animal Tracking as an Eye on Life and Planet’. Science 348 (6240): aaa2478–aaa2478. https://doi.org/10.1126/science.aaa2478.
Koons, David N., Marlène Gamelon, Jean-Michel Gaillard, Lise M. Aubry, Robert F. Rockwell, François Klein, Rémi Choquet, and Olivier Gimenez. 2014. ‘Methods for Studying Cause-Specific Senescence in the Wild’. Methods in Ecology and Evolution 5 (9): 924–33. https://doi.org/10.1111/2041-210X.12239.
Kuhn, Brian. 2005. ‘The Faunal Assemblages and Taphonomic Signatures of Five Striped Hyaena (Hyaena Hyaena Syriaca) Dens in the Desert of Eastern Jordan’. Levant 37 (1): 221–34. https://doi.org/10.1179/lev.2005.37.1.221.
Marescot, Lucile, Tavis D. Forrester, David S. Casady, and Heiko U. Wittmer. 2015. ‘Using Multistate Capture–Mark–Recapture Models to Quantify Effects of Predation on Age-Specific Survival and Population Growth in Black-Tailed Deer’. Population Ecology 57 (1): 185–97. https://doi.org/10.1007/s10144-014-0456-z.
Pompanon, Francois, Bruce E. Deagle, William O. C. Symondson, David S. Brown, Simon N. Jarman, and Pierre Taberlet. 2012. ‘Who Is Eating What: Diet Assessment Using Next Generation Sequencing’. Molecular Ecology 21 (8): 1931–50. https://doi.org/10.1111/j.1365-294X.2011.05403.x.
Rickett, Jennifer, Cody J. Dey, Jillian Stothart, Constance M. O’Connor, James S. Quinn, and Weihong Ji. 2013. ‘The Influence of Supplemental Feeding on Survival, Dispersal and Competition in Translocated Brown Teal, or Pateke (Anas Chlorotis)’. Emu - Austral Ornithology 113 (1): 62–68. https://doi.org/10.1071/MU12053.
Schaub, Michael, and Roger Pradel. 2004. ‘Assessing the Relative Importance of Different Sources of Mortality from Recoveries of Marked Animals’. Ecology 85 (4): 930–38. https://doi.org/10.1890/03-0012.
Sergio, Fabrizio, Alessandro Tanferna, Julio Blas, Guillermo Blanco, and Fernando Hiraldo. 2019. ‘Reliable Methods for Identifying Animal Deaths in GPS- and Satellite-Tracking Data: Review, Testing, and Calibration’. Journal of Applied Ecology 56 (3): 562–72. https://doi.org/10.1111/1365-2664.13294.
Smith, Lora L., David A. Steen, L. Mike Conner, and Jessica C. Rutledge. 2013. ‘Effects of Predator Exclusion on Nest and Hatchling Survival in the Gopher Tortoise’. The Journal of Wildlife Management 77 (2): 352–58. https://doi.org/10.1002/jwmg.449.
Tavecchia, Giacomo, Jaume Adrover, Antoni Muñoz Navarro, and Roger Pradel. 2012. ‘Modelling Mortality Causes in Longitudinal Data in the Presence of Tag Loss: Application to Raptor Poisoning and Electrocution’. Journal of Applied Ecology 49 (1): 297–305. https://doi.org/10.1111/j.1365-2664.2011.02074.x.
Tinker, M. Tim, Brian B. Hatfield, Michael D. Harris, and Jack A. Ames. 2016. ‘Dramatic Increase in Sea Otter Mortality from White Sharks in California’. Marine Mammal Science 32 (1): 309–26. https://doi.org/10.1111/mms.12261.
The relevance of trophic interactions to wild animal welfare
Luke Hecht explores the welfare implications of food chain length, biomass distribution, and predation.
Introduction
Almost all animals live off of energy ultimately derived from the sun by the photosynthetic activity of plants. This is true even of animals who do not eat plants as part of their own diet, but rather eat other animals who in turn ate plants. The transfer of energy from the bodies of individuals of one species to those of a different species is known as trophic interaction, or more colloquially, a food chain.
Figure 1. A simple food chain (Hui 2012).
According to many ethical views, an ecosystem supporting more happy animals is preferable to one which supports fewer equally happy animals. In many cases, communities with multi-level trophic chains support more animals than communities with simpler chains. But how additional trophic complexity influences the quality of animal lives is unclear — from an individual’s perspective, passing energy up the food chain requires their death and the loss of the opportunity to invest that energy in their own life or that of their offspring. To begin to address this apparent tradeoff, we will need to understand how trophic interactions influence the quality and quantity of animal lives in an ecosystem. In this article, I will specifically explore the welfare implications of food chain length, biomass distribution, and predation.
Figure 2. A more complex food chain, showing indirect effects of trophic interactions (Hui 2012).
Food chain length and stability
There is a directionality to food chains, which classically begin with plants at their base and end with an apex predator. These species are said to occupy different trophic levels. The number of trophic levels an ecosystem supports, and how diverse each one can be, is influenced by a plethora of factors. One such factor is the raw amount of energy available from lower trophic levels, known as productivity.
The energy derived from plants is expended in the biological activities of each organism it passes through, resulting in only a fraction of that energy being left over for use by higher trophic levels (Pimm 1988). After being sieved through a certain number of trophic levels, there may not be enough energy available to sustain an additional trophic level. At this point, the food chain is said to be energetically limited.
Many food chains are not limited by energy availability. Instead, some food chains terminate due to historical contingency. For example, in young ecosystems, there may be no immigrants of an appropriate species to continue the chain (Doi and Hillebrand 2019). Food chains may also end due to the unstable population dynamics that result when an additional trophic level is added to the system (Zhao et al. 2019). To understand why, consider a simple community composed of a predator, an herbivore, and a plant species. Herbivores consume plants, and are subsequently killed and eaten by predators. These trophic interactions influence the population dynamics of all three groups. For example, the addition of a predator to a simple herbivore-plant food chain might suppress the population of herbivores, in turn relieving some of the herbivory pressure on the plant population.
The indirect effect of the predator-prey interaction on the size of the plant population is known as a trophic cascade. Most of the time trophic interactions are stable, but they can be destabilizing, especially when there are many factors influencing population sizes. If the predator in this example was able to eradicate the herbivore, perhaps during an already low phase in their population cycle, the entire food chain might collapse, condemning the remaining predators to starvation.
There is a rich literature on the conditions required for stable predator-prey relationships, and destabilization is thought to be more likely when food chains are longer, leading to more cascading effects and more opportunities for the chain to be severed by the extinction of one species (Post 2002). However, theory and experiment also suggest that the destabilizing effect of food chain length can be compensated for by biodiversity at each level of the food chain, reducing the probability that an unstable interaction will sever the chain (Zhao et al. 2019). In this sense, food chains can be thought of as towers that become more susceptible to toppling with length (“vertical” diversity), and more resilient with breadth (“horizontal” diversity). Food chain length matters for wild animal welfare because destabilization through extinction of a particular species can lead to harmful outcomes such as starvation, unsustainable population growth, and intensified competition among remaining species (Ebenman et al. 2004), while trophic biodiversity can influence how many animals an ecosystem is able to support (Duffy et al. 2005).
Welfare implications of ecosystem biomass distribution
If all organisms in an ecosystem experienced equally positive welfare, then maximizing the productivity of the ecosystem would lead to higher total welfare. In reality, things are not so straightforward. Some individuals may suffer so substantially that their cumulative welfare over their lives is negative. Other organisms may contribute nothing to the welfare equation, as most of the biomass on our planet is made up of organisms that are unlikely to be sentient (such as microorganisms, plants, and fungi) or animals whose sentience and welfare capacity is difficult to ascertain (such as arthropods and nematodes) (Bar-on et al. 2018). Of course, self-sustaining populations of these organisms are instrumental to the ecosystem services that support happy, sentient animals.
Just as the quality of animals’ lives likely varies between species, so does the number of lives that can be sustained. Both factors influence the total amount of welfare in an ecosystem. Even among animals who are almost certainly sentient, including most vertebrates, there is extreme variation in average body mass and metabolic rate (Healy et al. 2019). These differences in energy use imply that more lives might be lived in an ecosystem composed of smaller or more metabolically efficient animals than an equally productive ecosystem composed of larger or less efficient animals, where the same amount of energy is divided among fewer individuals.
When animals die of things like disease, starvation, or accidents and are not immediately eaten by predators or animal scavengers, much of their biomass is used by microorganisms as the body is decomposed. Such decomposition adds additional layers of trophic interaction before a fraction of the originally available biomass reaches a sentient organism. The energy lost to microorganisms could be a net loss for wild animal welfare if the resource could have gone to improving the life of a sentient animal or extending an already positive life.
The concept of ecotrophic efficiency refers to the proportion of biomass of a particular species that is used and lost in the biological functioning of individuals of that species, or passed on to a higher trophic level via death by predation, relative to the energy that is lost via generalist decomposers. Causes of death other than predation generally lower a species’ ecotrophic efficiency. For example, Krebs et al. (2003) estimated the ecotrophic efficiencies of several species in the Canadian Arctic. They found that ecotrophic efficiency was high (~70%) among small herbivores such as lemmings and hares, who were predominantly killed by predators, but low (~9%) among large herbivores such as caribou and muskox. The concentration of biomass into high-welfare individuals is the ideal function of food chains in wild animal welfare.
A conflict of interest between predators and their prey
No animal wants to be killed by a predator, but no predator wants to die of starvation. The conflict of interest between predators and prey seems like a deadlock when framed this way, but the interested parties are not equal in size — a single predator generally needs to kill multiple prey animals to survive.
Trophic assimilation efficiency, or the conversion rate of prey biomass into predator biomass, is expected to average around 10-50%, with higher efficiencies when predators and prey are more similar in size and physiology (Sanders et al. 2016). To illustrate this point, suppose a predator consumes an animal whose body contains 100% of the energy the predator requires for the year. If the trophic assimilation efficiency is 25%, for instance, then the predator would need to consume 4 such animals per year to survive. Jensen and Miller (2001), for example, estimate that a wolf consumes 12-36 deer per year.
For a given assimilation efficiency, the greater the metabolic disparity between predator and prey, the more prey animals the predator will have to consume, as the energetic content of the prey animal would be a smaller proportion of the predator’s metabolic needs. Such disparities are common in marine and many terrestrial contexts, where predators tend to be larger than their prey (Andersen et al. 2008; Barnes et al. 2010). On the other hand, very high trophic assimilation efficiencies (~90%) have been reported among some insect parasitoids, where size and nutrient requirements of predator and prey are exceptionally well matched (Harvey et al. 2006).
If the lives of predators and prey were equal in length and welfare quality on average, trophic assimilation efficiency would be decisive, and any value less than 100% would mean that introducing a predator species to a population of herbivores would reduce the total welfare of the community. In practice, these assumptions probably rarely hold. A more nuanced calculation of the welfare consequences of predation needs to take into account the actual effect of predation on prey welfare, as well as differences in average lifetime welfare between predator and prey individuals.
Implications for welfare biology
One of the most important knowledge gaps for understanding the net welfare value of predation in a given community is whether predator-caused mortality is additive, compensatory, or depensatory with alternative causes of death. What would the life and death of a prey animal have been like otherwise? Answering this requires understanding demographic patterns of mortality and welfare for the population in question, such as the average age at which prey are killed. For example, Carroll (2013) found that the removal of wolves and bears from the vicinity of McGrath, Alaska resulted in a doubling of the proportion of newborn moose surviving to adulthood (>2 years), but had a negligible effect on adult life expectancy, implying that predation in this system mainly threatens juvenile moose who might have had long lives ahead of them. Research into predator-induced stress, density-dependent welfare, and the relative severity of alternative causes of death will also help us understand the net impact of predators on the lives of their prey.
The concepts of ecotrophic efficiency and trophic assimilation efficiency seem valuable to account for in welfare-focused ecological restoration and novel ecosystems. For example, under conditions where predation is thought to lead to better welfare outcomes overall, the ecosystems with highest net welfare might be those with high populations of small herbivores, whose biomass would be more efficiently transferred to high-welfare predators at the end of their lives. On the other hand, when predation is thought to be net-negative or where high-welfare animal scavengers are present, systems dominated by ecotrophically inefficient species such as larger herbivores could contain greater overall welfare. In contexts where human activity is already influencing ecosystem composition, it is worthwhile to consider these potential welfare consequences in our planning.
Trophic interactions, by distributing energy among animals, are part of the welfare value of maintaining functioning ecological communities. Sustaining multiple biodiverse trophic levels leads to a greater proportion of primary productivity ultimately being converted into sentient animals. The quality of life these animals naturally experience remains highly uncertain, and many ecosystems are already being perturbed by human activities in ways that are not calculated to improve wild animal welfare. However, further research into trophic efficiencies and the welfare of predator-prey systems can prepare us to restore ecosystems in ways that benefit many animals, not just a few.
Optimal population density: trading off the quality and quantity of welfare
Luke Hecht explains how, in some cases, reducing wild animals’ population densities by relatively small amounts might facilitate large increases in their welfare.
Introduction
Largely as a result of human development, the sizes of many wild animal populations have been falling.1 Much of this decline has resulted not from direct killing of wild animals by humans, but indirectly by fragmenting their habitats and cutting animals off from the resources that sustained their historical population sizes.2,3 This has immediate consequences for the welfare of individuals, who may face starvation, competition for resources, or conflict with humans as they try to access resources in their former habitat (e.g. urban pigeons4; fur seal5; black bear6). According to many ethical views, it is better to have more happy animals than fewer equally happy animals; but as a population grows, so does the number of individuals who may be adversely affected by further growth, requiring us to think carefully about trade-offs between the quantity and quality of welfare. A thorough understanding of individual welfare levels in a given population and how they are affected by changes in population density could eventually enable us to estimate the population size which would maximize the welfare experienced in that population.
Population modeling and density-dependence
The density of a population is defined by the ratio between the number of individuals and the amount of habitat or a limiting resource available to them; if a population grows or its habitat declines, population density increases. The population size (N) at which a balance between birth rate and death rate is achieved—its maximum sustainable size—is known as the “carrying capacity” (K) under given environmental conditions (Box 1).
Box 1
A population’s growth rate per generation (r) is equal to the difference between the number of births (b) and the number of deaths (d): r = b - d. The growth rate before any effects of density are taken into account is denoted r0. To the extent that r0 exceeds zero, density-dependent effects must reduce age-specific survival or fecundity rates to achieve a stable population. The population size (N) at which r = 0 and the population remains stable long-term is known as the carrying capacity (K).
These parameters come together in the logistic growth equation, where any excess of births over deaths shrinks to zero as N approaches K: dn/dt = r0N(K - N)/(K).
While this equation captures how extremes of population density relate to population growth rates, it is certainly incomplete. For example, intermediate densities may have less consistent effects than the simple logistic function suggests, especially in populations facing strong density-independent stressors. Populations inhabiting an environment that changes much more rapidly than the time between their generations may never reach their carrying capacity at any given time, but may still encounter strong density effects during periods when N > K, as might occur during a drought or similar period of low productivity.
Death rates may rise and birth rates may fall as a result of stressors experienced by the animals in a population. These stressors can be density-dependent or density-independent. For example, competition for food can lead to starvation and limit the energy available for reproduction7, and crowding in early life can cause an animal to age prematurely8. Infectious diseases may also spread more rapidly in a dense population9.
Other causes of mortality are density-independent. In a river, water temperature or pollutants might kill fish at a rate that is independent of the number of fish in the river10. Indeed, density-independent factors can make an environment so hostile that the maximum stable population size is very low, and the surviving individuals face no meaningful competition for resources.
Whether a certain factor is density-dependent or density-independent can also vary according to the circumstances. For example, the direct threat posed to any individual by fierce winds and lightning during a storm is independent of density, but density-dependent mortality might follow in the wake of the storm if a limiting resource has been damaged. Population density within a certain range can also have positive effects for individual welfare by providing safety in numbers and more accessible social interactions. For example, having fellow newts around during their transition to maturity means that individuals of Triturus cristatus are less likely to undertake dangerous migrations to find mates11.
Welfare implications of population density
In a recent article, I proposed the concept of welfare expectancy as a way to link age-specific survival rates and welfare. Welfare expectancy is a sum of the lifetime welfare an individual born into a given population can expect to experience. Age-specific survival rates may also be correlated with age-specific welfare, suggesting that any density-related impairment of survival could greatly reduce welfare expectancy, especially when it affects juvenile animals.
A general expectation of declining welfare expectancy per individual as a population grows towards its carrying capacity raises the possibility that the population size which maximizes the total welfare of a population may be smaller than its maximum sustainable size. Whether this occurs depends on how many individuals the population would contain at its carrying capacity and how average welfare varies with population size (Box 2). The conditions are most likely to be met if the strongest negative effects of population density on welfare manifest at high densities (i.e., as N approaches K; Figure 1). This could occur under the following three conditions:
- if lower densities primarily limit reproduction while higher densities affect survival rates.
- if density-dependent mortality disproportionately affects older animals at lower densities but young animals at high densities; or
- if density-independent effects are nearly sufficient to stabilize the population’s growth rate, so any negative effects of density are only felt near the carrying capacity.
In general, the shape of average welfare expectancy with respect to density depends on the particular ecology and life history of the population in question, especially whether density preferentially affects fecundity versus survival rates or the survival rates of young versus old individuals. If increased density is mainly accommodated by reduced fecundity or late-life survival, for instance, then average welfare might not decline so steeply as a population grows. Theory and reviews of demographic models for a variety of large vertebrates suggest that juvenile survivorship is usually the first casualty of high population densities12,13,14, which is one set of conditions under which we might expect the welfare-optimal population density to be lower than the population’s carrying capacity. Part of the explanation for this pattern in large vertebrates is the competitive advantage older animals typically have over younger animals who are smaller and less experienced. However, it is not yet known how broadly these age dynamics apply among the much more numerous smaller-bodied, “fast-living” animals, where there are examples of adult advantage15 and disadvantage16. More research is needed into the expected shape and mechanisms of density-dependence among their populations.
Box 2
Let WN represent the average welfare expectancy at population size N and let K represent the population size at carrying capacity. Then, the welfare-optimal population size will be less than the carrying capacity if WK-1/WK > K/(K - 1).
The welfare expectancy per individual in a population depends on the life expectancy from birth and the welfare they typically experience over that lifespan. If average welfare is positive, average welfare expectancy will correlate with life expectancy. If an increase in population density disproportionately harms the youngest animals, this can greatly reduce life expectancy.
Implications for welfare interventions
If we are completely uncertain about the absolute welfare level of animals in a population, then it follows that we are completely uncertain about whether their lives are dominated by pleasure or suffering. If their lives contain more goodness on average, then increasing the population size would be expected to increase the total amount of welfare experienced within the population as a whole. On the other hand, if their lives are primarily unhappy , then adding more unhappy individuals through population growth would actually subtract from the population’s total welfare. From a standpoint of complete uncertainty, then, the expected value of population growth or decline is symmetrical17. Whether or not population growth increases or decreases total welfare of the population depends on the balance of the positive and negative experiences of the individuals added by that growth.
However, the existence of a density-dependent decline in average welfare would set up an asymmetry in favor of relatively smaller populations, since increasing population density would reduce the probability that average welfare remains net-positive, giving population growth a lower expected value than population reduction. Of course, this heuristic assumes complete ignorance of the population’s welfare characteristics, which is rarely the case.
On the other hand, if we knew the exact average welfare expectancy for all possible population sizes (WN), we could predict its optimal density and also define a ‘margin of error’ on either side within which total welfare expectancy would be expected to at least equal that obtained at carrying capacity (Figure 1). This could provide a wide target for interventions aiming to improve welfare by managing population density. Notably, the largest range and scope for improvement in total welfare over what would occur naturally is possible when the most aversive effects of population density only manifest at high densities. This suggests that a review of evidence on the shapes of density-dependence curves for various species could indicate how impactful managing around population density might be as a form of welfare intervention.
Figure 1. Left: Hypothetical curves depict how average (per-individual) welfare expectancy might decline with increasing population density, depending on whether the most aversive effects of density develop at low densities (“early” in population growth), at a constant rate (“continuous”), or only at high population densities (“late”). Right: Average welfare under each of these three scenarios is multiplied by population density to give total welfare. Total welfare below the level achieved at the maximum sustainable size (K) is shaded. Despite average welfare always being positive, the welfare-optimal population size (O) is less than the maximum sustainable population size under the “late” (O=0.75) and “continuous” (O=0.81) scenarios. Total welfare continues to increase up to K under the “early” model (O=1.00), where higher population densities have a smaller effect on average welfare.
Figure 2. Left: As the population grows from 0 to K average welfare declines from net-positive to net-negative (red shading). A population of wild animals might appear to have very poor welfare at their natural population density, yet have the potential for good welfare at lower population density. Right: In such a case, their total welfare would be maximized by sustaining a medium-sized population, with “Late” density effects increasing the population’s optimal size and potential welfare.
Population density could be managed (and any effects ameliorated) by reducing birth rates, culling individuals from the existing population, or increasing resource availability. Culling is currently the default method for managing animal densities, usually targeting those considered “pests.” Although welfare is occasionally considered, this approach is suboptimal because it involves inflicting suffering on some animals and cutting their lives short (even though culling methods may be less painful than natural causes of death18). Non-lethal reduction of population density could be achieved through fertility control, directly limiting the number of animals born without shortening the lives of extant individuals. A complementary approach is reducing the effective density of a population by increasing the quantity or quality of its habitat using the principles of restoration ecology. This could reduce density and improve welfare in the short-term without requiring any reduction in population size. However, without additional measures artificially limiting their growth rate, populations would simply return to their natural sizes and any associated harmful effects of density, so to be sustainable long-term this would still need to be paired with fertility control or another method. A combination of fertility control and habitat improvement could form the basis of a welfare-focused approach to wildlife management. Such an approach must also take into account non-target impacts on other species before being put into practice19.
Conclusions
It is currently technologically infeasible to make population management decisions that consider the needs of every individual wild animal. We therefore need to determine guiding principles to help us predict the likely results of various population management projects, even under uncertainty about the absolute level of welfare the relevant animals experience. Understanding the density-dependence of welfare in a given population provides one such principle. Furthermore, given sufficient information about absolute welfare levels, this approach could also be used to identify a window of effect sizes where an intervention is expected to increase total welfare relative to the natural long-term size of a population.
The effects of varying levels of density on survival and reproductive rates are exceedingly difficult to determine for any population, given the generational timescales involved and the fact that any population density other than K must by definition be unstable without outside intervention. As a result, precise estimates of density-dependence are rare in the literature, though there is empirical and theoretical support for certain generalizations. Direct evidence on wild animal welfare is also lacking, though we can make inferences from survival rates, body condition and behavior. Research in both of these areas could be high-impact, as an understanding of how welfare expectancy varies with population size would be crucial for planning many large-scale wild animal welfare interventions.
References
-
World Wildlife Federation. 2018. Living Planet Report - 2018: Aiming Higher. ↩
-
Andren, H. (1994). Effects of habitat fragmentation on birds and mammals in landscapes with different proportions of suitable habitat: a review. Oikos, 355-366. ↩
-
Ripple, W. J., Wolf, C., Newsome, T. M., Hoffmann, M., Wirsing, A. J., & McCauley, D. J. (2017). Extinction risk is most acute for the world’s largest and smallest vertebrates. Proceedings of the National Academy of Sciences, 114(40), 10678-10683. ↩
-
Senar, J. C., Montalvo, T., Pascual, J., & Peracho, V. (2017). Reducing the availability of food to control feral pigeons: changes in population size and composition. Pest management science, 73(2), 313-317. ↩
-
Doidge, D. W., Croxall, J. P., & Baker, J. R. (1984). Density‐dependent pup mortality in the Antarctic fur seal Arctocephalus gazellu at South Georgia. Journal of Zoology, 202(3), 449-460. ↩
-
Murphy, S. M., Augustine, B. C., Ulrey, W. A., Guthrie, J. M., Scheick, B. K., McCown, J. W., & Cox, J. J. (2017). Consequences of severe habitat fragmentation on density, genetics, and spatial capture-recapture analysis of a small bear population. PloS one, 12(7), e0181849. ↩
-
McMahon, C. R., Harcourt, R. G., Burton, H. R., Daniel, O., & Hindell, M. A. (2017). Seal mothers expend more on offspring under favourable conditions and less when resources are limited. Journal of Animal Ecology, 86(2), 359-370. ↩
-
Nettle, D., Monaghan, P., Gillespie, R., Brilot, B., Bedford, T., & Bateson, M. (2015). An experimental demonstration that early-life competitive disadvantage accelerates telomere loss. Proceedings of the Royal Society B: Biological Sciences, 282(1798), 20141610. ↩
-
Civitello, D. J., Allman, B. E., Morozumi, C., & Rohr, J. R. (2018). Assessing the direct and indirect effects of food provisioning and nutrient enrichment on wildlife infectious disease dynamics. Philosophical Transactions of the Royal Society B: Biological Sciences, 373(1745), 20170101. ↩
-
Boulenger, C., Acou, A., Gimenez, O., Charrier, F., Tremblay, J., & Feunteun, E. (2016). Factors determining survival of European eels in two unexploited sub‐populations. Freshwater Biology, 61(6), 947-962. ↩
-
Cayuela, H., Schmidt, B. R., Weinbach, A., Besnard, A., & Joly, P. (2019). Multiple density‐dependent processes shape the dynamics of a spatially structured amphibian population. Journal of Animal Ecology, 88(1), 164-177. ↩
-
Eberhardt, L. L. (1977). "Optimal" Management Policies for Marine Mammals. Wildlife Society Bulletin, 162-169. ↩
-
Gaillard, J. M., Festa-Bianchet, M., & Yoccoz, N. G. (1998). Population dynamics of large herbivores: variable recruitment with constant adult survival. Trends in Ecology & Evolution, 13(2), 58-63. ↩
-
Bergman, E. J., Doherty, P. F., White, G. C., & Holland, A. A. (2015). Density dependence in mule deer: a review of evidence. Wildlife Biology, 21(1), 18-30. ↩
-
Figler, M. H., Cheverton, H. M., & Blank, G. S. (1999). Shelter competition in juvenile red swamp crayfish (Procambarus clarkii): the influences of sex differences, relative size, and prior residence. Aquaculture, 178(1-2), 63-75. ↩
-
Fletcher, W. J. (1988). Intraspecific interactions between adults and juveniles of the subtidal limpet, Patelloida mufria. Oecologia, 75(2), 272-277. ↩
-
Briggs, R. A., "Normative Theories of Rational Choice: Expected Utility", The Stanford Encyclopedia of Philosophy (Fall 2019 Edition), Edward N. Zalta (ed.). ↩
-
Bradshaw, E. L., & Bateson, P. (2000). Welfare implications of culling red deer (Cervus elaphus). Animal Welfare, 9(1), 3-24. ↩
-
Clarke, M., & Ng, Y. K. (2006). Population dynamics and animal welfare: issues raised by the culling of kangaroos in Puckapunyal. Social Choice and Welfare, 27(2), 407-422. ↩
Age-specific survivorship frames the expected value of wild animal welfare
This research note is an extension of Luke Hecht’s paper, The importance of considering age when quantifying wild animals’ welfare, which was published in Biological Reviews in December 2021. Luke introduces “welfare expectancy” as a framework for summing up the different levels of well-being animals might experience over the course of their lives, helping to compare the welfare consequences of interventions and natural pressures that may disproportionately affect animals of particular ages.
This project is a continuation of work that began at Animal Ethics, which is covered here. We thank them for their collaboration.
As part of an ongoing project to understand the welfare of wild animals, I analyzed age-specific survivorship as it relates to welfare, introducing a new concept for understanding the lives of wild animals: welfare expectancy. Welfare expectancy can serve as a framework for weighing up the different levels of well-being animals might experience over the course of their lives, helping to model the welfare consequences of interventions and natural pressures, such as predation, that may disproportionately affect animals of particular ages.
Wild animals’ lives are extraordinarily diverse. Individuals of different species occupy different habitats, consume different resources, and engage in different behaviors. Even within species, their fortunes vary depending on their circumstances of birth and exposure to chance events which lead to differential survival, mating success, and welfare.
Organisms must make trade-offs between their own survival and reproduction, and the survival of their offspring. These trade-offs evolve to maximize lifetime reproductive output, which defines an individual’s fitness. However, it is crucial to recognize that fitness and welfare are not the same. For example, a strategy which maximizes the average fitness of offspring could lead to reproductive success in adulthood for a few, but short lives for most.
In order to identify safe and tractable ways to improve wild animals' lives, welfare biology needs to identify the major threats to their welfare. In the face of the diversity of individual experiences wild animals may have, we need a way to tally them up in order to assess the overall welfare of a population. Expected value does this by taking the sum of the value of each outcome multiplied by its probability. For example, to calculate the life expectancy of a population (i.e. the expected value of lifespan), one would multiply the proportion of individuals who die at a certain age by the number of years they lived and sum this across all possible lifespans.
In my forthcoming paper (previous version), I introduce a similar concept of “welfare expectancy” to formalize the relationship between age-specific survivorship and lifetime welfare. Consider a species for which welfare is poor in early life, but high in adulthood. If the probability of surviving early life is high, then the lifetime expected value of welfare for an individual born into that population may be high, because most individuals are going to have a chance to live out their best years in adulthood. If the probability of surviving early life is low, then most individuals will only live to experience the juvenile period of poor welfare. Conversely, in a species where welfare is higher in early life than in adulthood (e.g. due to good parental care), the net welfare of even a short-lived animal could be relatively high.
We are profoundly uncertain about whether most animals’ lives are dominated by pleasure or suffering, or even how to go about weighing these up. Therefore, it may be prudent to concentrate on a measure of “relative welfare expectancy” (RWE), representing the normalized welfare expectancy of a population divided by its life expectancy. For a fixed life expectancy, the highest welfare expectancy is achieved by maximizing the proportion of animals living to experience the best years of life while minimizing the proportion experiencing the worst years, as illustrated in Figure 1.
Figure 1: Assuming a fixed maximum lifespan, relative welfare expectancy is maximized when the majority of individuals live through ages characterized by above-average welfare. For example, in the “bad” case, the highest-welfare periods come late in life; most individuals in such a population only experience the poorer-welfare early ages.
As part of an investigation of age-specific mortality jointly supported by Animal Ethics and Wild Animal Initiative, I analyzed published demographic models for 152 populations of 88 species to survey patterns of age-specific mortality and used them to illustrate the implications of age-specific welfare. The vast majority of animals live very short lives; not only in absolute terms, but also relative to the longest-lived members of their species. In fact, of the populations analyzed, average lifespans were on average 16% of a species’ maximum lifespan, with only 5% of populations having life expectancies >33% of their maximum (Figure 2). Importantly, this represents an average across populations, not across individuals. Because short-lived species tend to be more populous, lives in nature are likely to be cut short far more often than these numbers suggest. Depending on how welfare varies with age in their respective species, especially short-lived individuals will be missing out on a great deal of positive and/or negative experience.
Figure 2: Histogram of life expectancy as a proportion of the species’ theoretical lifespan. Most animals live only a small fraction of their potential lives, even in species where the theoretical lifespan is already relatively short.
Confident application of the welfare expectancy concept will require empirical data on values of age-specific welfare, which are currently scarce for wild animal populations. A plausible working hypothesis, however, is that the average day-to-day welfare experienced by an animal of a given age is proportional to their probability of surviving that period of life. The justification for this is that the same factors which lead to mortality (e.g. disease, vulnerability to predators, competition for food) have been shown to lead to chronic stress and poor physical condition.
Although we do not know where to draw the line between net-positive and net-negative welfare for any species, under this hypothesis the probability that welfare over a given period is net positive would also be proportional to the survival probability. For example, a year in which an animal has a 60% chance of surviving might be twice as likely to be net-positive as a year which they have a 30% chance of surviving. In the absence of evidence, for the purposes of this report we decided to represent age-specific welfare exclusively as a positive number ranging from 0 to 1 (equal to the survival rate), for illustrative purposes only. Readers are free to adjust the welfare expectancy values given in Table A1 of the report according to where they feel the line between net-positive and net-negative welfare may lie for any particular species by subtracting the product of its life expectancy and the minimum annual survival rate they feel would correspond to net-positive welfare.
For an animal to have an enjoyable life on net, they must experience enough pleasure to compensate for the pain of their death. Cause of death, and therefore the duration and pain of an animal’s experience of dying, may also vary with age similarly to welfare. In a hypothetical species, juveniles might be most likely to starve while adults are most likely to be predated, with the relative probabilities of these and other mortality factors shifting over a lifetime. If the pain of death is a sufficiently strong factor to negate some of the positive welfare an animal might have experienced while alive, age-specific variation in the incidence of various manners of death and their severity would also be important to account for. (See Michael Plant and Brian Tomasik for contrasting views on this.)
At the individual level, welfare expectancy unites two distinct concepts: day-to-day quality of life and the quantity of welfare experienced over an individual’s lifetime. However, a similar quantity-quality distinction applies at the level of populations, with welfare expectancy addressing the quality side of the argument and quantity being determined by the population size. Ideally, a population should be managed in such a way that maximizes its total welfare expectancy. A predictive understanding of population ecology is therefore crucial for contextualizing information on wild animal welfare, as well as evaluating and prioritizing among interventions that differentially affect various age or stage groups. The welfare expectancy framework might serve as an early paradigm for welfare biology.